Jean-Martin Charcot first described ‘La sclérose en plaques disséminées’ and its associated neurologic triad - the combination of nystagmus, intention tremor and scanning speech - in 1868. Today, we know this condition to be Multiple Sclerosis (MS), a chronic inflammatory disease of the central nervous system affecting 2.5 million individuals worldwide. Histopathology reveals it is characterized by focal demyelinating lesions in the brain and spinal cord, with a clinical presentation that is dependent on the CNS regions affected.
The introduction of imaging in the second half of the 20th century enabled pathologic lesions to be detected in vivo. MRI in particular, with its superior contrast over CT, conceded greater sensitivity to the disease than clinical assessments alone. Quantitative and qualitative evaluation of MS was possible for the first time. MRI findings now form an essential component of the 2017 MAGNIMS/McDonald criteria for diagnosing MS and are key to tracking disease progression and responses to novel therapies.
The Challenges of White Matter Lesion Segmentation
Manual labeling of MRI images remains the most accurate method for MS white matter lesion segmentation (WMLS). But this process can considerably infringe upon a radiologist’s workload. Lesion loads vary from high to low, sizes from a few mm3 to cm3, and shapes from ovoid to irregular. Studies place the average time for the task to be performed on a single 3D scan as high as 50 minutes! [1]
What’s more, manual segmentation results are error-prone and susceptible to high inter- and intra-rater variability, intensified by differences in the scanner, acquisition sequence, and image quality. Such shortcomings have prompted the development of automated methods for MS WMLS, several of which are available in the QMENTA cloud platform and delineated below.
MS White Matter Lesion Segmentation in the QMENTA platform
MRI-based MS lesion assessment typically utilizes hyperintensities in T2 FLAIR images. QMENTA’s LST-LPA 2.0 Workflow requires only a single FLAIR input to output estimated lesion maps, counts, and volumes. It implements SPM’s LST toolbox in the form of the lesion prediction algorithm (LPA) [2]. A large-scale logistic regression model alongside data from 53 cases of severe MS was used to train the algorithm. Its use has been validated across scanners and even in other diseases, such as Alzheimer’s. A longitudinal pipeline (Longitudinal Lesion Analysis) is also offered where different time points are available to facilitate assessments of lesion dissemination throughout both space and time.
LPA finds further utility in lesion-filling methods. Cortical atrophy is accelerated in MS patients and is shown to correlate with measures of clinical disability, but volume estimates can be biased in brains with high lesion loads. The Lesion Segmentation Workflow uses a lesion-filling approach to replace the lesion intensities derived via LPA performed on FLAIR and thus generates a T1 image that can be more accurately segmented thereafter.
Deep learning algorithms have also been recently suggested for WMLS, though with generally inferior results compared to human raters. In 2020 QMENTA partnered with mediaire to integrate their CE-marked Lesion Characterization module, among others. Although deep learning-based, they show that their algorithms are in fact on par with neuroradiologists in terms of segmentation accuracy [3]. Their 2D U-Net-like architecture is trained on axial, sagittal, and coronal FLAIR slices, subsequently employing a unanimous voting model which only accepts lesions that are present in all three orientations. Accepted lesions are then classified by region according to MAGNIMS/McDonald criteria to enable differentiation of MS lesions from age-related hyperintensities. The mediaire method ranked 1st in MICCAI 2021’s MSSEG-2 challenge [4].
We at QMENTA have developed our own deep learning-based tool [5], with the added feature of an in-app editor designed to further improve segmentation accuracy in FLAIR images: Q-White Matter Hyperintense Lesion Segmentation W/ Editor.
The prestige of supervised approaches is somewhat weakened by their poor generalizability. By nature, the relationship between images and labels in the training set is directly encoded into the algorithm, and for this reason, results may be limited when applied to images acquired with different scanners/protocols.
To side-step this caveat, the Lesion TOADS Workflow utilizes an unsupervised classifier [6]. Here lesions are modeled as an additional tissue class that shares its spatial distribution prior with healthy white matter. This takes place within the context of a topology-preserving atlas-based framework, which essentially enables simultaneous whole-brain and MS lesion segmentation of the combined T1-FLAIR input. It bypasses the two-phase approach necessary with lesion filling, making it one of the few dedicated tools for characterizing atrophy patterns in MS.
Finally, we introduce FreeSurfer’s SAMSEG [7] to the QMENTA platform. This tool completely decouples computational models of anatomy from models of the imaging process to accept a wide variety of hardware, protocols, and sequences, including pre-and post-contrast T1 images. Simultaneous whole-brain and MS WMLS are possible through the augmentation of its generative model with a binary lesion map. 41 brain structures are segmented (versus Lesion TOADS’ 10), with robust results even in controls. Further, MS lesion maps may be edited for false positives and negatives via a platform-based viewer.
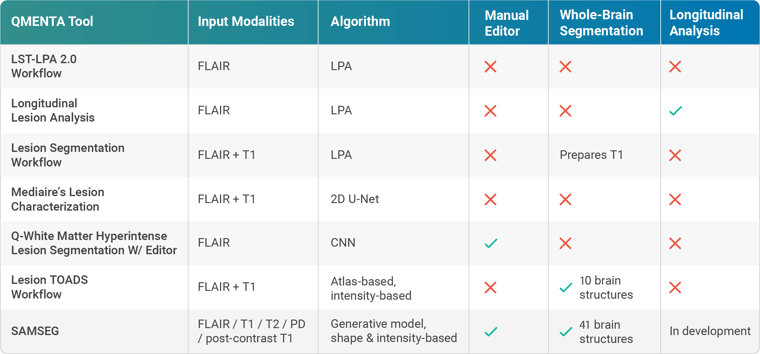
References:
[1] Storelli, L., Pagani, E., Rocca, M. A., Horsfield, M. A., Gallo, A., Bisecco, A., Battaglini, M., De Stefano, N., Vrenken, H., Thomas, D. L., Mancini, L., Ropele, S., Enzinger, C., Preziosa, P., & Filippi, M. (2016). A Semiautomatic Method for Multiple Sclerosis Lesion Segmentation on Dual-Echo MR Imaging: Application in a Multicenter Context. AJNR. American Journal of Neuroradiology, 37(11), 2043–2049.
[2] Paul Schmidt. Bayesian inference for structured additive regression models for
large-scale problems with applications to medical imaging. PhD thesis, Ludwig Maximilians-Universität München, January 2017.
[3] Hitziger, S., Ling, W. X., Fritz, T., D'Albis, T., Lemke, A., & Grilo, J. (2022). Triplanar U-Net with lesion-wise voting for the segmentation of new lesions on longitudinal MRI studies. Frontiers in neuroscience, 16, 964250.
[4] https://portal.fli-iam.irisa.fr/msseg-2/
[5] Puch, Santi & Sanchez, Irina & Hernàndez, Aura & Piella, Gemma & Prčkovska, Vesna. (2019). Global Planar Convolutions for improved context aggregation in Brain Tumor Segmentation. arXiv:1908.10281
[6] Shiee, N., Bazin, P. L., Ozturk, A., Reich, D. S., Calabresi, P. A., & Pham, D. L. (2010). A topology-preserving approach to the segmentation of brain images with multiple sclerosis lesions. NeuroImage, 49(2), 1524–1535.
[7] Cerri, S., Puonti, O., Meier, D. S., Wuerfel, J., Mühlau, M., Siebner, H. R., & Van Leemput, K. (2021). A contrast-adaptive method for simultaneous whole-brain and lesion segmentation in multiple sclerosis. NeuroImage, 225, 117471.
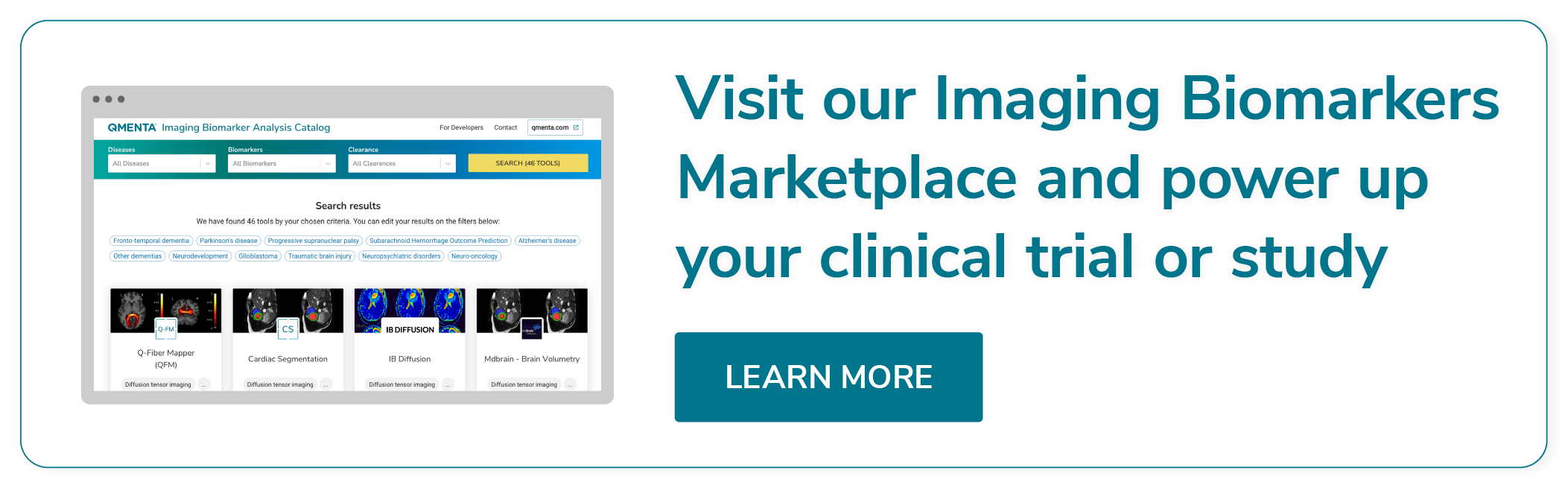